In business landscape, efficient data management is essential for making informed and strategic decisions. Two predominant approaches to data integration are ETL (Extract, Transform, Load) and ELT (Extract, Load, Transform). While both aim to process and consolidate information from multiple sources, they differ in the sequence of their stages and how they handle data. Understanding these differences is crucial for determining which strategy best suits your company’s specific needs.
ETL (Extract, Transform, Load)
The ETL process consists of three main stages:
- Extraction: Data is gathered from multiple sources, including databases, CRM systems, and flat files.
- Transformation: Extracted data is cleaned and converted to meet the standards and requirements of the target system. This may include data cleansing, business rule application, and format conversion.
- Loading: Finally, the transformed data is loaded into a data warehouse, making it available for analysis and reporting.
ETL is traditionally used in environments where data must be highly structured and of high quality before storage. This approach is common in on-premises systems and scenarios where data volumes are not excessively large. Additionally, ETL is ideal when transformations are complex and require strict control over data quality before loading.
ELT (Extract, Load, Transform)
ELT reverses the last two steps of ETL:
- Extraction: Similar to ETL, data is extracted from various sources.
- Loading: Raw data is loaded directly into the target system, typically a data lake or a cloud-based data warehouse.
- Transformation: Once in the target system, data is transformed as needed for specific analyses.
This approach has become more viable with the rise of cloud storage solutions, which offer scalable processing and storage capabilities. ELT is particularly useful for handling large volumes of unstructured data and provides greater flexibility for analysis since transformations occur on demand. Additionally, ELT is well-suited for businesses with powerful data warehouses looking for faster integration, especially in big data environments.
Key differences between ETL and ELT
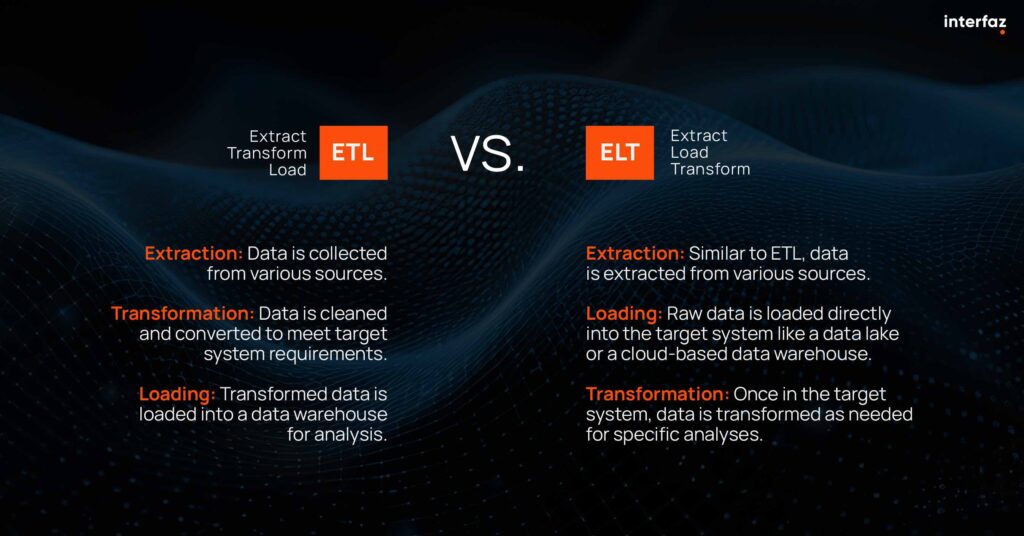
- Processing sequence: In ETL, data is transformed before being loaded into the target system, whereas in ELT, transformation occurs after loading.
- Infrastructure: ETL is typically implemented in on-premises environments or systems with storage and processing limitations, requiring data preparation in advance. ELT leverages the massive processing capabilities of cloud systems, allowing large volumes of data to be loaded without prior transformation.
- Flexibility and scalability: ELT offers greater flexibility in analyzing data in different formats and is more scalable due to the elastic nature of cloud resources.
- Processing time: ELT can significantly reduce loading times, as data is first loaded and then transformed, taking advantage of the target system’s processing power.
Performance and efficiency
Performance is a critical factor when selecting between ETL and ELT. ETL processes transformations before loading, which can be time-consuming, especially with large data volumes.
On the other hand, ELT leverages the processing power of modern destination systems, such as cloud-based data warehouses, to execute transformations faster after loading. This enables faster data ingestion and more agile preparation for analysis. However, it is crucial to ensure that the target system has the necessary processing capacity to handle transformations without affecting overall performance.
Security and data governance
Security and data governance are fundamental considerations in any integration strategy. ETL, by transforming data before loading, ensures thorough cleansing and validation, allowing only high-quality data into the target system. This reduces the risk of introducing incorrect or corrupted data into the data warehouse.
In contrast, ELT loads raw data directly into the target system, which can pose security challenges if proper controls are not in place. Establishing robust data governance policies is essential to ensure that only authorized users access and transform data according to defined guidelines.
Current trends in data integration
Technological advancements and evolving business needs have shaped current data integration trends:
- Cloud integration: With the widespread adoption of cloud solutions, ELT has gained popularity due to its ability to handle large data volumes and perform transformations within powerful cloud data warehouses.
- Hybrid integration: Some organizations are adopting hybrid approaches that combine the best of ETL and ELT—performing critical transformations before loading and deferring others until later, leveraging the target system’s processing capabilities.
- Automation and advanced tools: The use of advanced tools that automate ETL and ELT processes is increasing, enabling companies to optimize data workflows and reduce manual errors.
Use cases for ETL and ELT
The choice between ETL and ELT depends significantly on specific use cases:
- ETL: Ideal for regulated industries such as banking and healthcare, where data quality and compliance are critical. For example, a financial institution that needs to consolidate transaction data from multiple systems for regulatory reporting would benefit from the control and precision ETL offers.
- ELT: Suitable for companies handling large volumes of unstructured data requiring real-time analytics. For instance, an e-commerce company analyzing customer behavior data to personalize offers in real time would benefit from ELT’s flexibility and scalability.
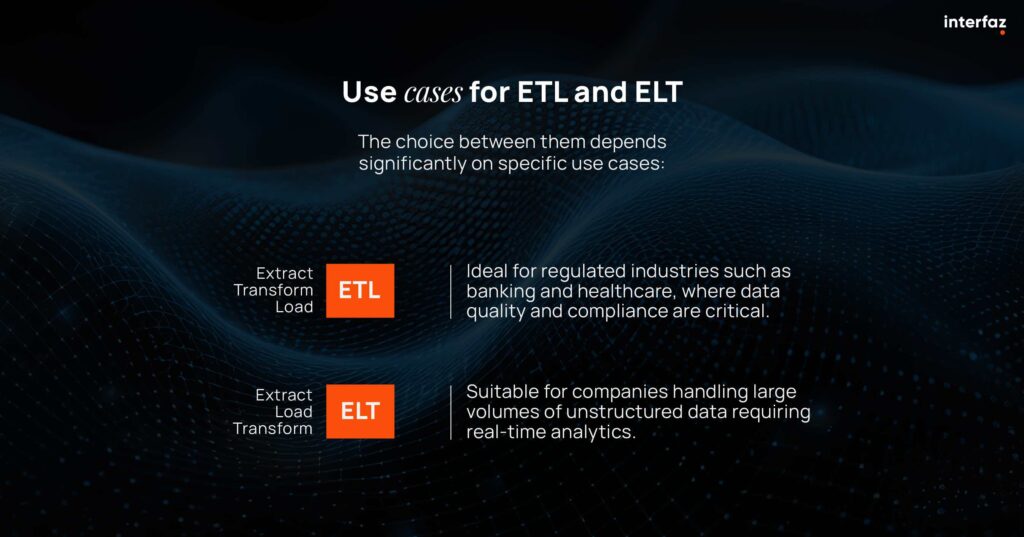
Factors to consider when choosing between ETL and ELT
The choice between ETL and ELT depends on various factors:
- Data volume and type: ELT is more suitable for large volumes of unstructured data, while ETL is preferable for structured data requiring high quality before analysis.
- Available infrastructure: If your company operates on cloud infrastructure and requires scalability, ELT is a viable option. In on-premises environments with limited resources, ETL may be more appropriate.
- Analysis requirements: If rapid and flexible raw data analysis is needed, ELT is beneficial. If analysis depends on pre-cleaned and structured data, ETL is more suitable.
- Transformation complexity: If data transformations are complex and require detailed control, ETL may be the best choice.
- Security and compliance: ETL provides a more secure process, ensuring cleaner data and reducing the risk of data encoding errors.
Leading ETL and ELT tools in 2025
As technology evolves, so do ETL and ELT tools. Below are some of the most notable tools on the market:
- Apache NiFi: An open-source ETL tool that efficiently processes and distributes data. It features an intuitive user interface and is ideal for real-time data flows.
- Stitch: An open-source ELT data integration platform offering self-service ELT and automated pipelines. It supports data extraction from over 130 platforms, services, and applications.
- Airbyte: A leading open-source ELT platform with the largest catalog of data connectors (350+ and growing). It integrates with dbt for data transformation and with Airflow for workflow orchestration.
- Fivetran: A data integration platform that provides pre-built connectors for a wide range of data sources. It is known for its ease of use and ability to handle large datasets.
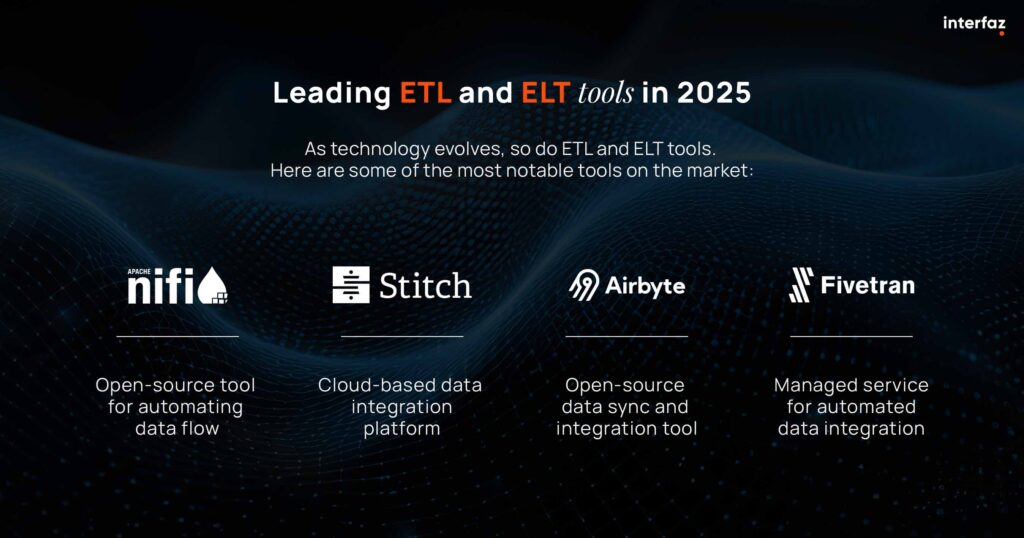
Conclusion
The decision between ETL and ELT is not one-size-fits-all; it should be based on your company’s specific needs, available infrastructure, and data analysis objectives. ETL offers rigorous control and is suitable for environments where data quality is paramount. ELT provides flexibility and scalability, leveraging the processing capabilities of modern cloud systems. By carefully evaluating these factors and considering current trends, your company can implement a data integration strategy that optimizes operations and facilitates informed, strategic decision-making.
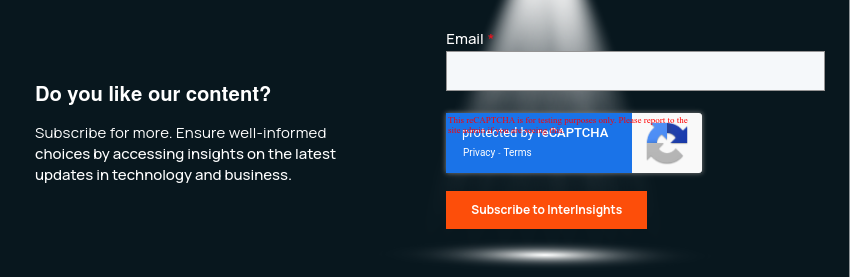