In today’s digital world, using predictive analytics is crucial for companies to stay competitive. In this blog, we’ll explore how using Machine Learning in predictive analytics is changing different industries. We’ll talk about the real benefits it brings, the common challenges companies face, the important tools and platforms available, and the ethical and privacy issues to consider.
1. Benefits of implementing Machine Learning in business predictions:
Using ML in predictive analytics has clear benefits for businesses. According to McKinsey, companies using ML can boost their operating margin by up to 45%. This happens because ML helps streamline processes, cut down on waste, and make operations more efficient overall.
Additionally, machine learning can provide a significant competitive advantage by predicting demand more accurately in the sales sector. Research from HBR found that companies using ML to forecast demand experience a 20% reduction in inventory costs and a 25% improvement in forecast accuracy.
In marketing, can help companies better understand their audience and personalize marketing strategies. Using ML to segment their audience more effectively and tailor campaigns, resulting in higher conversion rates and customer loyalty. According to Forbes, companies using ML to segment their audience experience a 20% increase in conversion rates and a 10% reduction in customer acquisition costs.
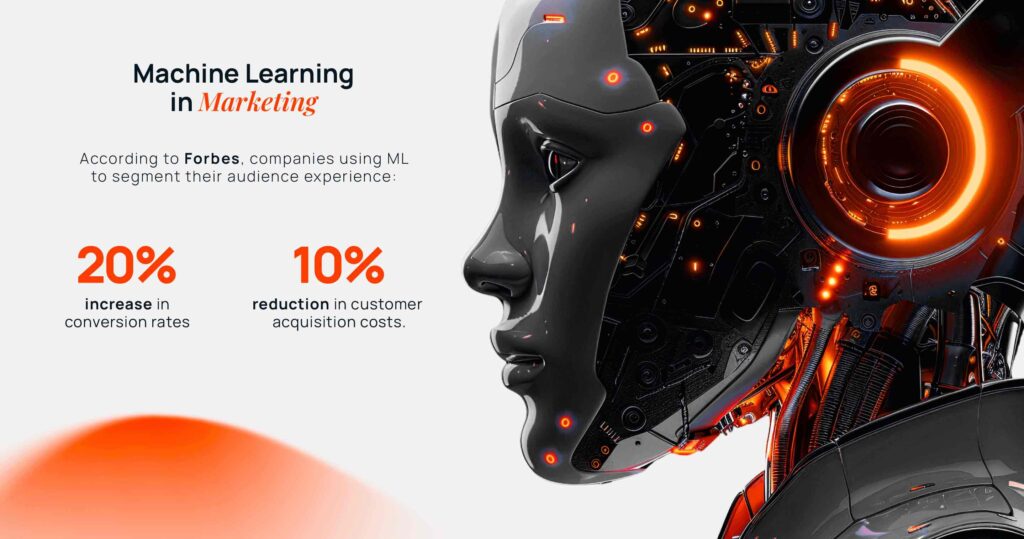
2. Challenges and considerations:
Despite the evident benefits, companies face significant challenges when implementing machine learning solutions. One of the most significant challenges is data quality. It’s estimated that 80% of the time spent on ML projects is spent on data preparation and cleaning. Ensuring that the data used to train models is accurate, complete, and representative is crucial for project success.
Selecting the right algorithms is also crucial. Different problems require different approaches, and choosing the wrong algorithm can lead to ineffective models and inaccurate predictions.
Understanding results can pose a challenge. ML models often prove complex, particularly for individuals lacking technical expertise. Ensuring clarity in decision-making processes and effectively communicating results are crucial to encourage the utilization of ML solutions within the company.
Finally, the successful implementation requires an organizational culture that values experimentation and continuous learning. Companies must be willing to invest in training and skill development for their staff, as well as establish processes and policies that encourage interdepartmental collaboration and innovation.
3. Tools and platforms for Machine Learning:
Fortunately, there are numerous tools and platforms available to facilitate the implementation of machine learning solutions. TensorFlow, an open-source library developed by Google, is one of the most popular and widely used tools in the ML community. TensorFlow offers a wide range of functionalities, from building and training models to deployment in production and monitoring.
Other options include scikit-learn, a Python machine learning library that offers a variety of algorithms and tools for data analysis and predictive modeling; Amazon SageMaker, a fully managed ML platform in the cloud that simplifies the process of building, training, and deploying machine learning models; and Google Cloud AI Platform, which offers a range of services and tools for developing and deploying machine learning models on Google Cloud.
In addition to these tools, there are a variety of libraries and frameworks specific to different ML apps and use cases. For example, Keras and PyTorch are popular among researchers and practitioners of deep learning, while H2O.ai focuses on scalable and high-performance machine learning solutions.
It’s important to note that choosing the right tool or platform will depend on the specific requirements of the project, such as the size and complexity of the data, the availability of computational resources, and the team’s expertise.
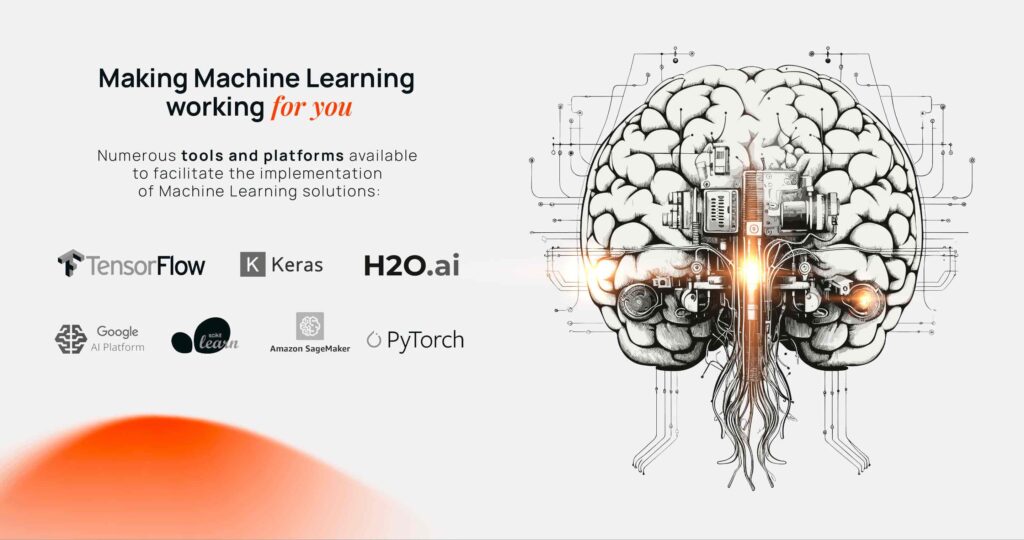
4. Ethical and privacy considerations:
Companies using machine learning in decision-making need to tackle ethical and privacy worries. For instance, algorithmic bias is a rising problem when models learn from biased data. To lessen this, using fair data and testing rigorously to fix biases is vital.
Data privacy violation is also a significant concern. It’s crucial for companies to implement robust data privacy practices and comply with regulations such as the European Union’s General Data Protection Regulation (GDPR) to ensure responsible use of machine learning and protect individuals’ privacy.
In addition to complying with data privacy regulations, companies should also consider the ethical impact of their algorithmic decisions. This includes assessing how their ML models could affect different groups of people and taking steps to mitigate any negative impacts. For example, some companies have implemented algorithm audits to identify and correct potential biases in their machine learning models.
Conclusion
Machine learning provides many chances to improve predictive analytics in businesses. It helps make predictions more accurate, cuts costs, and finds new market opportunities. But it’s also important to deal with challenges like making sure data is good, picking the right methods, and thinking about ethics and privacy.
By leveraging available tools and platforms and adopting an ethical and responsible approach, companies can unlock the full potential of machine learning in predictive analytics.
At Interfaz, we understand the importance of machine learning in predictive analytics. Our team is committed to helping businesses implement innovative and effective solutions that drive growth and competitiveness. If you’re ready to take the next step towards more advanced predictive analytics, contact us. We’re here to be your trusted partner.
Sources:
- McKinsey, “The State of Machine Learning”
- Harvard Business Review, “How Machine Learning Is Revolutionizing Sales Forecasting”
- Harvard Business Review, “The Problem with AI”
- Forbes, “The Impact of Machine Learning on Marketing”
- Forbes, “Why Machine Learning Projects Fail”